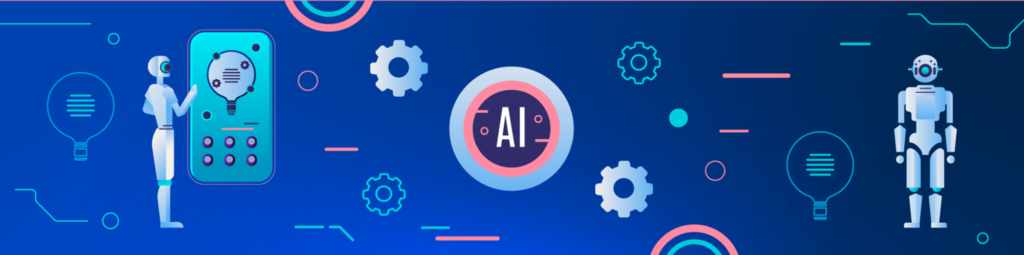
AI Trends and Predictions
1. Generative AI and Creative Applications
- Trend: Generative AI, including tools like ChatGPT and DALL-E, has become mainstream. These models can create text, images, videos, and even 3D models, opening new possibilities in content creation, art, marketing, and design.
- Prediction: Generative AI will continue to evolve, with more advanced models capable of creating highly realistic simulations, personalized content, and assisting with complex creative tasks. This trend may lead to new industries and workflows, especially as tools improve in customization and controllability.
2. AI in Healthcare and Life Sciences
- Trend: AI is making strides in healthcare, particularly in diagnostics, drug discovery, and personalized medicine. AI-driven models can analyze medical images, predict disease risk, and even recommend treatment options.
- Prediction: AI will likely become deeply integrated into clinical workflows, leading to faster, more accurate diagnoses and personalized treatment plans. In the near future, we may see AI applications in real-time patient monitoring, predictive health, and perhaps even robotic-assisted surgeries.
3. Increased Focus on Explainable AI (XAI)
- Trend: With AI systems being deployed in high-stakes fields, explainable AI is gaining attention. Users, especially in sectors like finance, healthcare, and law enforcement, require transparency and interpretability to trust AI decisions.
- Prediction: Explainable AI models and tools will become more advanced, allowing users to understand decision-making processes in real-time. We may see regulations mandating interpretability for high-risk AI, as well as advancements in tools that make complex models like neural networks more interpretable.
4. Enhanced AI Model Efficiency and Eco-Friendly AI
- Trend: Training large AI models is resource-intensive, leading to concerns about environmental impact. Recently, there has been a focus on making AI models more efficient and less resource-demanding.
- Prediction: AI models will become more efficient, with advancements in techniques like transfer learning, model compression, and edge computing. This will help reduce the environmental impact and bring powerful AI capabilities to smaller devices, making it more accessible and sustainable.
5. AI and Cybersecurity
- Trend: Cybersecurity threats are increasing, and AI is playing a crucial role in detecting and mitigating these threats. AI-driven systems can identify anomalies, detect patterns in large datasets, and respond faster than traditional systems.
- Prediction: The cybersecurity sector will see more advanced AI tools that can proactively identify and counteract sophisticated threats. AI-driven cybersecurity may also become more accessible to smaller businesses, helping protect critical data and infrastructure at all levels.
6. Real-Time Language Translation and NLP Improvements
- Trend: Natural language processing (NLP) is evolving rapidly, with models achieving near-human levels of language understanding. Real-time translation and AI chatbots have improved greatly, creating smoother interactions across languages.
- Prediction: NLP will become even more nuanced, supporting real-time, highly accurate translations with context-specific understanding. This could lead to more seamless international collaboration and the integration of NLP-driven customer service chatbots across industries.
7. AI-Driven Personalization and Customer Experience
- Trend: Companies are increasingly using AI to create personalized customer experiences in e-commerce, entertainment, and marketing. AI algorithms analyze user behavior to deliver tailored recommendations, advertisements, and content.
- Prediction: Personalization will become more sophisticated, with AI systems able to predict customer preferences more accurately and in real-time. This may lead to hyper-personalized services and experiences, particularly in retail, media, and digital marketing.
8. AI Ethics, Fairness, and Responsible AI
- Trend: As AI adoption grows, there’s a heightened focus on the ethical implications of AI, including bias, privacy, and accountability. Governments and organizations are beginning to establish frameworks to ensure ethical AI use.
- Prediction: Regulatory frameworks like the EU AI Act will set new standards, particularly for high-risk applications. Companies will likely need to conduct regular AI audits, bias assessments, and ensure transparency. Ethical AI practices may become a core part of corporate strategy, similar to sustainability practices today.
9. AI and the Internet of Things (IoT) Integration
- Trend: AI and IoT are becoming more intertwined, creating smarter and more autonomous devices. AI processes data collected from IoT devices to enable automation and real-time insights in homes, cities, and industries.
- Prediction: With 5G expanding IoT capabilities, we’ll likely see AI-powered IoT systems revolutionize sectors like transportation (smart traffic systems), manufacturing (predictive maintenance), and agriculture (precision farming). These integrations may bring about highly efficient, automated environments.
10. AI-Driven Automation in the Workplace
- Trend: AI is automating repetitive tasks in sectors like manufacturing, finance, and customer service, improving productivity and reducing human error.
- Prediction: The next wave of AI-driven automation will impact knowledge work, potentially transforming roles in fields like data analysis, project management, and even writing. New job categories focused on AI oversight, interpretation, and customization will likely emerge, balancing job displacement concerns with opportunities for upskilling.
11. AI’s Role in Education and Learning
- Trend: AI is starting to reshape education through personalized learning platforms, virtual tutors, and automated grading.
- Prediction: As AI tools improve, they’ll become integral to educational environments, supporting adaptive learning paths tailored to individual student needs. AI may also assist teachers in identifying struggling students early and providing customized resources.
12. Quantum Computing and AI
- Trend: Quantum computing holds the potential to revolutionize AI, particularly in areas requiring vast computational power. Though still in early stages, there’s a growing interest in the intersection of quantum computing and AI.
- Prediction: In the next decade, quantum computing may significantly accelerate AI’s ability to solve complex problems, from climate modeling to drug discovery. Although practical applications are years away, the combination of AI and quantum computing could unlock possibilities we can’t yet fully envision.
13. AI for Environmental Sustainability
- Trend: AI is being used to tackle environmental challenges, from optimizing energy usage in data centers to monitoring deforestation with satellite imagery.
- Prediction: Sustainability-focused AI applications will expand, with AI helping to optimize renewable energy grids, manage natural resources, and monitor pollution. AI may become a critical tool in combating climate change as global efforts intensify.
In summary, AI is becoming more powerful, ethical, efficient, and pervasive across industries. These trends highlight the potential of AI to solve complex problems, though challenges in ethics, regulation, and sustainability will require careful attention as we move forward.
Industry-specific AI applications
1. Healthcare
- Diagnostics: AI models like computer vision are used to analyze medical images (e.g., MRI, CT scans) to detect diseases such as cancer and fractures.
- Personalized Medicine: Machine learning algorithms predict treatment responses based on individual genetics, leading to more effective personalized care.
- Virtual Health Assistants: AI-driven chatbots and virtual assistants answer patient queries, provide reminders, and support telemedicine, improving accessibility.
2. Finance
- Fraud Detection: AI models analyze transaction data to detect unusual patterns that might indicate fraudulent activities.
- Algorithmic Trading: AI analyzes market data and executes trades at optimal times, helping to maximize profits.
- Customer Service Automation: Chatbots and virtual assistants in banking provide 24/7 support for common customer queries and transactions.
3. Retail
- Recommendation Systems: AI-powered recommendation engines (like those used by Amazon or Netflix) analyze purchase behavior to suggest products, boosting sales.
- Inventory Management: Predictive analytics help forecast demand, optimize stock levels, and reduce waste.
- Customer Insights and Personalization: AI tools analyze consumer behavior to create personalized shopping experiences through targeted marketing.
4. Manufacturing
- Predictive Maintenance: Sensors and AI models monitor machinery health to predict failures, minimizing downtime and repair costs.
- Quality Control: Computer vision systems identify product defects on assembly lines in real-time, ensuring high quality.
- Supply Chain Optimization: AI optimizes inventory levels, logistics, and supplier relationships for efficiency and cost savings.
5. Transportation & Logistics
- Autonomous Vehicles: AI enables self-driving cars, trucks, and drones, which could transform freight and last-mile delivery.
- Route Optimization: AI algorithms help logistics companies optimize delivery routes, saving fuel and reducing delivery times.
- Traffic Management: AI is used in smart cities to manage traffic signals, reduce congestion, and improve emergency response times.
6. Energy
- Smart Grids: AI helps optimize energy distribution in real-time based on demand patterns, improving grid stability.
- Renewable Energy Forecasting: Machine learning models forecast wind or solar output, making renewable energy integration more reliable.
- Energy Efficiency: AI enables building automation systems to adjust lighting, heating, and cooling based on occupancy and weather, saving energy.
7. Agriculture
- Precision Farming: AI-powered drones and sensors gather data on soil quality, moisture levels, and crop health for targeted interventions.
- Yield Prediction: Machine learning predicts crop yields based on weather and soil data, aiding in planning and resource allocation.
- Pest and Disease Control: AI models analyze crop images to detect pests or diseases early, enabling timely intervention.
8. Education
- Personalized Learning: AI-powered platforms adjust learning content and pace based on each student’s progress and needs.
- Automated Grading: Natural language processing (NLP) can be used to grade essays and short answers, freeing up educators’ time.
- Learning Analytics: AI analyzes student performance data to identify struggling students early and provide targeted support.
9. Real Estate
- Property Valuation and Analysis: AI models analyze market trends, location data, and property features to provide accurate valuations.
- Virtual Tours and Customer Service: AI-powered virtual tours allow prospective buyers to explore properties remotely, and chatbots provide instant support.
- Smart Buildings: AI enables real-time monitoring and management of building systems, improving efficiency and tenant satisfaction.
10. Entertainment and Media
- Content Personalization: Streaming platforms use AI to recommend shows and movies based on viewer behavior.
- Content Creation: AI can assist in generating scripts, music, or even complete media projects, providing creative support to content creators.
- Audience Analytics: AI helps analyze audience behavior and feedback, guiding content production and marketing strategies.
Each industry is leveraging AI uniquely to solve specific challenges, boost efficiency, and improve customer experiences, making it a transformative technology across sectors.
AI Ethics and Regulations
1. Bias and Fairness
- Challenge: AI models can perpetuate or even amplify existing biases in data, leading to discriminatory outcomes. This is especially problematic in sectors like hiring, criminal justice, and finance, where biased AI decisions can have serious consequences.
- Solutions: Addressing bias requires careful dataset curation, bias-detection algorithms, and techniques like fairness constraints in model training. Transparency about training data and model limitations is also crucial.
- Regulatory Approach: Some jurisdictions, like the EU, are working on regulations mandating AI audits and fairness checks to prevent discriminatory practices.
2. Transparency and Explainability
- Challenge: AI systems, especially complex deep learning models, can function as “black boxes,” making it hard to understand how they arrive at certain decisions. This lack of explainability can be a barrier to trust, especially in high-stakes areas like healthcare or finance.
- Solutions: Developing explainable AI (XAI) techniques that allow insights into model decision-making processes is an active area of research. Visualization tools, feature importance metrics, and simpler model structures are commonly used.
- Regulatory Approach: The EU AI Act, for example, requires transparency for high-risk AI systems, mandating clear explanations of AI-based decisions to affected users.
3. Privacy and Data Protection
- Challenge: AI systems often require large volumes of data, which raises privacy concerns, particularly in surveillance, healthcare, and social media applications.
- Solutions: Techniques like data anonymization, federated learning (where data remains on user devices), and differential privacy help reduce privacy risks.
- Regulatory Approach: GDPR in the EU sets strict rules around personal data usage, impacting how AI models can collect and process information. The act includes provisions for data minimization, informed consent, and individuals’ rights over their data.
4. Accountability and Responsibility
- Challenge: When AI systems make errors or cause harm, it’s often unclear who is responsible: the developer, the user, or the company deploying the AI?
- Solutions: Building AI models with rigorous testing, validation, and continuous monitoring helps reduce the risk of harmful outcomes. Ensuring clear accountability structures within organizations is also important.
- Regulatory Approach: Policies are emerging that focus on holding both developers and users accountable for AI deployment. The EU AI Act, for instance, proposes requirements on high-risk AI systems that include accountability documentation and ongoing oversight.
5. Safety and Reliability
- Challenge: Safety is critical in applications such as autonomous vehicles, healthcare diagnostics, and industrial robotics, where AI failures could lead to accidents or harm.
- Solutions: AI safety research focuses on model robustness, ensuring that systems can handle unexpected inputs without failing or causing harm. Rigorous testing under varied conditions is essential.
- Regulatory Approach: Regulations are being developed to enforce strict safety standards, such as requiring testing in realistic environments for self-driving cars and medical devices.
6. Job Displacement and Economic Impact
- Challenge: Automation through AI could displace jobs, particularly in sectors like manufacturing, retail, and transportation, impacting workers and local economies.
- Solutions: Reskilling and upskilling initiatives are essential to help workers transition to new roles. Governments and organizations can also consider complementary policies like universal basic income (UBI) or targeted support for affected workers.
- Regulatory Approach: Some governments are exploring policies to manage AI-driven job transitions, such as funding for retraining programs and incentives for companies to retain human workers in AI-assisted roles.
7. Environmental Impact
- Challenge: Training large AI models requires substantial energy, contributing to carbon emissions. This is especially true for AI models like deep neural networks, which can be resource-intensive.
- Solutions: Techniques like model optimization, green computing practices, and using renewable energy for data centers can reduce AI’s carbon footprint.
- Regulatory Approach: There is increasing pressure for companies to report on and minimize the environmental impact of AI. Future regulations may enforce carbon offsets or energy efficiency standards for data centers and large-scale AI projects.
8. Ethical AI Development and Deployment
- Challenge: AI applications, such as deepfakes or surveillance technology, can be used for unethical purposes, raising questions around AI’s role in society.
- Solutions: Ethical AI development focuses on designing AI in line with human values, promoting responsible AI use, and preventing misuse. Companies and developers often adhere to ethical guidelines or principles, though these vary widely.
- Regulatory Approach: Regulations like the EU AI Act categorize AI applications by risk level, banning or strictly regulating certain “high-risk” applications, like AI used in surveillance or social scoring.
9. Human Rights and Autonomy
- Challenge: AI systems could potentially infringe on human rights, especially in authoritarian regimes where AI is used for mass surveillance or social scoring.
- Solutions: Human-centered AI aims to ensure that technology respects human rights, freedoms, and autonomy. Transparency, privacy, and individual control over data are foundational aspects.
- Regulatory Approach: There is a strong push, particularly in democratic countries, to regulate AI in ways that protect citizens’ rights. For example, the EU’s AI Act includes strict controls on mass surveillance technologies and prohibits social scoring.
10. Global Governance and Collaboration
- Challenge: Different countries have varying views on AI ethics and regulation, making it challenging to develop a unified approach. Some countries prioritize innovation, while others emphasize ethical safeguards.
- Solutions: International bodies like the OECD and UNESCO are creating frameworks and guidelines to foster ethical AI development globally. Collaborative efforts across borders can harmonize standards and encourage ethical AI practices.
- Regulatory Approach: International collaborations, like the Global Partnership on AI, are working to establish shared principles, and more countries are joining initiatives to create cohesive AI governance frameworks.